The energy sector is undergoing a transformative shift. As renewable energy adoption accelerates and grid complexity increases, traditional cloud-dependent systems struggle to deliver the speed and precision required for modern power management. Edge computing in energy has emerged as a critical solution, enabling real-time decision-making directly at the source—solar farms, wind turbines, and substations. By processing data locally, edge systems eliminate the delays of cloud-based architectures, allowing energy providers to detect issues instantly, balance energy flows dynamically, and integrate distributed resources seamlessly.
This article explores how edge computing in energy, combined with IoT analytics, is redefining grid operations. We’ll examine its technical foundations, highlight key applications, and demonstrate why this technology is essential for utilities, renewable operators, and industrial energy systems.
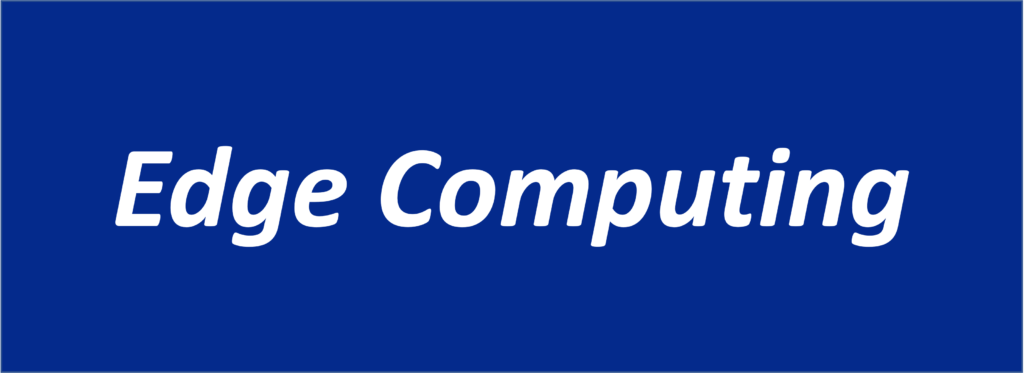
Table of contents
Challenges of Driving Edge Adoption
Modern energy grids face critical challenges that demand decentralized solutions:
- Renewable Energy Variability: Fluctuations in solar and wind generation destabilize voltage and frequency.
- Aging Infrastructure: Legacy systems struggle to manage bidirectional power flows from distributed energy resources.
- Cybersecurity Risks: Centralized cloud architectures create vulnerabilities for cyberattacks.
- Data Overload: Massive volumes of sensor data overwhelm traditional processing systems.
Edge computing addresses these issues by embedding intelligence directly into grid assets, enabling faster, localized responses.
How Edge Computing Transforms Energy Management
Edge systems analyze data in real time, enabling actions that were previously unattainable:
- Instant Fault Detection and Response
- Localized Analytics: Edge devices use machine learning to identify anomalies like overheating equipment or line faults.
- Autonomous Isolation: Systems can isolate affected grid segments almost instantaneously, preventing widespread outages.
- Predictive Insights: Sensors detect early signs of equipment wear, enabling timely maintenance.
Example: Solar farms use edge AI to identify electrical irregularities in photovoltaic panels, preventing potential hazards and maintaining consistent energy output.
- Dynamic Load and Frequency Control
- Real-Time Coordination: Edge systems balance energy flows between rooftop solar, batteries, and EV chargers.
- Frequency Stabilization: During sudden drops in renewable generation, edge controllers reroute stored energy to maintain grid stability.
- Demand Optimization: Algorithms adjust industrial energy use during peak periods, lowering operational costs.
- Enhanced Cybersecurity
- On-Device Security: Edge gateways encrypt data locally, minimizing exposure to external breaches.
- Zero-Trust Protocols: Continuous verification of devices and commands blocks unauthorized access.
Key Components of an Edge-Enabled Energy System
A robust edge infrastructure relies on four core elements:
- Edge IoT Devices
- Smart Sensors:
- Monitor grid conditions like voltage, current, and temperature with high precision.
- Track equipment health through vibration and thermal analysis.
- Edge Computing Gateways:
- Aggregate data from diverse protocols (Modbus, DNP3, IEC 61850).
- Run lightweight AI models for real-time insights.
- Built to withstand harsh environments, including extreme temperatures and humidity.
- Hybrid Communication Networks
- 5G Networks: Prioritize critical data transmission with ultra-low latency.
- Time-Sensitive Networking (TSN): Synchronize data streams across grid nodes for deterministic performance.
- Edge-to-Cloud Architecture
- Edge Layer: Handles urgent tasks like fault isolation and load balancing.
- Cloud Layer: Supports long-term analytics, model training, and grid simulations.
- Energy-Specific Software
- Grid Management Platforms: Orchestrate distributed energy resources and automate fault recovery.
- Cybersecurity Suites: Detect threats in real time and enable secure remote updates.
Applications: Edge Computing in Action
- Wind Farm Maintenance
- Challenge: Equipment failures cause costly downtime.
- Edge Solution:
- Sensors monitor turbine components for early signs of wear.
- Local AI models trigger maintenance alerts before failures occur.
- Adjust turbine operations to reduce stress on vulnerable parts.
- Outcome: Minimized unplanned outages and extended equipment lifespan.
- Urban Microgrid Efficiency
- Challenge: High energy demand strains local grids during peak hours.
- Edge Solution:
- Predict energy demand using real-time data from smart meters.
- Automatically shift loads to onsite batteries or solar panels.
- Optimize EV charging schedules to avoid overloads.
- Outcome: Reduced peak demand charges and increased renewable energy utilization.
- Industrial Power Quality
- Challenge: Heavy machinery generates harmful electrical distortions.
- Edge Solution:
- Analyze power quality metrics locally to detect distortions.
- Activate filters to neutralize disturbances in real time.
- Outcome: Improved equipment reliability and compliance with power standards.
- Solar Farm Safety
- Challenge: Electrical faults in solar arrays pose fire risks.
- Edge Solution:
- Detect irregular electrical patterns using edge AI.
- Disconnect faulty components immediately to prevent hazards.
- Outcome: Enhanced safety and reduced operational risks.
The Future of Edge Computing in Energy
Emerging innovations will expand edge capabilities:
- Self-Healing Grids: Autonomous systems that reroute power and resolve faults without human intervention.
- Collaborative AI: Edge devices across regions will share insights to improve grid resilience.
- Advanced Security: Next-generation encryption will safeguard edge networks from evolving threats.
Conclusion
Edge computing in energy is the foundation of modern, resilient power systems. By embedding intelligence directly into grid assets, energy providers can stabilize networks, integrate renewables seamlessly, and mitigate cybersecurity risks.