The global manufacturing landscape is undergoing a seismic shift. Traditional assembly lines, once reliant on manual labor and isolated machinery, are evolving into interconnected, intelligent ecosystems. This transformation, driven by Industry 4.0, is not merely about automation—it’s about creating self-optimizing systems that learn, adapt, and innovate. At the heart of this revolution lie three critical technological pillars: intelligent connectivity, edge-powered intelligence, and data-driven autonomy. Together, these pillars bridge the gap between “making” and “smart-making,” empowering manufacturers to achieve unprecedented efficiency, agility, and sustainability.
This article explores how these technologies converge to redefine modern manufacturing, offering actionable insights for businesses ready to embrace the future.
Table of contents
- The Challenges of Traditional Manufacturing
- Pillar 1: Intelligent Connectivity – The Nervous System of Smart Factories
- Pillar 2: Edge-Powered Intelligence – Brains at the Source
- Pillar 3: Data-Driven Autonomy – The Self-Sustaining Factory
- Applications – Industry 4.0 in Action
- The Future of Smart Manufacturing
- Conclusion
The Challenges of Traditional Manufacturing
Before delving into solutions, it’s essential to understand the limitations holding back traditional factories:
- Fragmented Systems: Machines, sensors, and software often operate in silos, creating data bottlenecks.
- ReactiveMaintenance: Equipment failures lead to costly unplanned downtime.
- Limited Adaptability: Rigid production lines struggle to accommodate custom orders or rapid design changes.
- Energy Inefficiency: Poor resource utilization drives up costs and environmental impact.
These challenges demand a holistic approach—one that integrates cutting-edge technologies into every layer of operations.
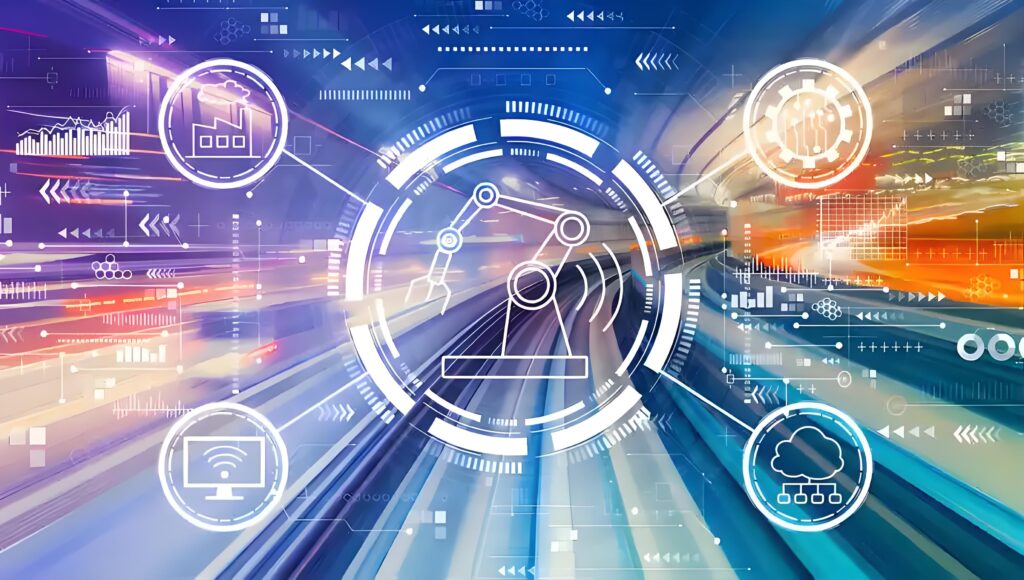
Pillar 1: Intelligent Connectivity – The Nervous System of Smart Factories
The first pillar of Industry 4.0 is intelligent connectivity, which ensures seamless communication between machines, humans, and systems.
5G & Time-Sensitive Networking (TSN)
- Ultra-Reliable Communication: 5G’s low latency and high bandwidth enable real-time coordination of robotic arms, AGVs, and IoT sensors. For example, in automotive assembly lines, 5G ensures welding robots and conveyor belts operate in perfect sync.
- TSN Integration: Time-sensitive networking guarantees prioritized data delivery for critical tasks, such as emergency machine shutdowns or precision calibration.
Industrial IoT Gateways
- Multi-Protocol Support: Gateways bridge legacy PLCs with modern cloud platforms, enabling data flow between Modbus and MQTT devices.
- RTK Positioning: High-precision positioning systems guide autonomous forklifts and drones in warehouses, minimizing navigation errors.
Impact: Factories achieve end-to-end visibility, from raw material intake to quality control, while reducing communication lag.
Pillar 2: Edge-Powered Intelligence – Brains at the Source
The second pillar Industry 4.0 , edge-powered intelligence, brings computational power directly to the factory floor, enabling instant decision-making.
Edge Computing Nodes
- Real-Time Analytics: Edge devices process sensor data locally, detecting anomalies like motor vibrations or temperature spikes. In semiconductor manufacturing, this allows immediate adjustments to etching processes.
- AI-Driven Quality Control: Cameras with embedded AI inspect products for defects, flagging issues without cloud dependency.
Adaptive Machine Learning
- Self-Optimizing Systems: Edge AI models learn from production patterns, optimizing equipment settings for energy efficiency. For instance, injection molding machines adjust pressure and temperature autonomously to reduce material waste.
Impact: Factories shift from reactive troubleshooting to proactive optimization, slashing downtime and waste.
Pillar 3: Data-Driven Autonomy – The Self-Sustaining Factory
The third pillar, data-driven autonomy, transforms factories into self-governing ecosystems capable of end-to-end optimization.
Unified AIoT Platforms
- Centralized Dashboards: Integrate data from machines, ERP systems, and supply chains into a single interface. Production managers monitor KPIs like OEE (Overall Equipment Effectiveness) in real time.
- Predictive Maintenance: AI analyzes historical data to forecast equipment wear, scheduling repairs before failures occur.
Closed-Loop Automation
- Self-Healing Systems: When a CNC machine detects tool wear, it automatically orders replacements and adjusts machining parameters.
- Dynamic Resourcing: Algorithms reallocate energy, labor, and materials based on shifting priorities—ideal for handling rush orders or supply chain disruptions.
Impact: Manufacturers achieve true autonomy, where systems self-optimize to meet quality, cost, and sustainability targets.
Applications – Industry 4.0 in Action
Automotive Manufacturing: Agile Production for Customization
Challenge: Automotive factories face mounting pressure to deliver personalized vehicles (e.g., custom interiors, EV configurations) without slowing production. Legacy systems struggle with frequent retooling and quality inconsistencies.
Solution:
- 5G-Enabled Flexible Lines:
- Autonomous mobile robots (AMRs) with RTK positioning navigate dynamically between workstations, delivering parts for bespoke vehicle builds.
- 5G-connected welding robots adjust parameters in real time based on material thickness data from IoT sensors.
- Edge-Powered Quality Assurance:
- AI cameras inspect paint finishes and weld seams, comparing results against digital twins of ideal specifications.
- Defects trigger automatic adjustments in polishing robots or rework queues.
Outcome:
- Seamless shifts between gas-powered, hybrid, and EV production on the same line.
- Reduced rework costs and faster delivery of customized orders.
Electronics Assembly: Zero-Defect Micro-Manufacturing
Challenge: Microscopic defects in circuit boards or semiconductor chips can lead to costly recalls. Traditional optical inspection systems miss subtle flaws.
Solution:
- AI-Augmented Microscopy:
- Edge AI analyzes high-resolution images from microscopes, detecting soldering cracks as narrow as a few micrometers.
- Self-learning algorithms improve detection accuracy by studying historical defect patterns.
- Closed-Loop Process Control:
- Real-time feedback from inspection systems adjusts soldering temperatures or component placement pressure.
- Digital twins simulate thermal stress impacts on circuit longevity.
Outcome:
- Near-perfect defect detection rates, minimizing warranty claims.
- Accelerated production of miniaturized components for IoT devices and wearables.
Heavy Machinery: Sustainable and Resilient Operations
Challenge: Energy-intensive processes like metal forging consume excessive power, while unplanned downtime disrupts project timelines.
Solution:
- Predictive Energy Optimization:
- Edge analytics correlate furnace temperature, material hardness, and energy consumption to identify waste.
- AI schedules high-energy tasks during off-peak utility pricing windows.
- Autonomous Health Management:
- Vibration sensors on hydraulic presses predict bearing failures weeks in advance.
- Self-diagnosing CNC machines order replacement parts automatically via integrated supply chain platforms.
Outcome:
- Lower carbon footprints aligned with ESG goals.
- Extended machinery lifespan through condition-based maintenance.
Pharmaceutical Production: Compliance at Scale
Challenge: Strict regulatory requirements demand flawless documentation, while contamination risks threaten batch integrity.
Solution:
- Blockchain-Enabled Traceability:
- IoT sensors track temperature, humidity, and airflow in cleanrooms, recording data on tamper-proof blockchain ledgers.
- Autonomous drones sanitize production areas based on real-time particle counts.
- AI-Driven Compliance Audits:
- Natural language processing (NLP) cross-references production logs against FDA/EU regulations, flagging discrepancies.
- Digital work instructions guide technicians via AR glasses, ensuring protocol adherence.
Outcome:
- Faster regulatory approvals and audit readiness.
- Elimination of human error in critical processes.
The Future of Smart Manufacturing
The next phase of Industry 4.0 will see even deeper integration of these pillars:
- Digital Twins: Virtual replicas of factories simulate production changes before implementation.
- Collaborative AI: Machines across global facilities share insights to improve efficiency collectively.
- Sustainable Autonomy: AI balances production goals with renewable energy usage and carbon targets.
Conclusion
The journey from “made” to “smart-made” hinges on mastering three pillars: intelligent connectivity, edge-powered intelligence, and data-driven autonomy. By adopting these technologies, manufacturers unlock:
- Resilience: Adapt swiftly to market shifts and disruptions.
- Efficiency: Minimize waste and maximize resource utilization.
- Innovation: Accelerate R&D cycles through data-backed insights.
The factories of tomorrow are not just automated—they’re alive with intelligence.